Machine learning in healthcare: Revolutionizing patient care and decision making
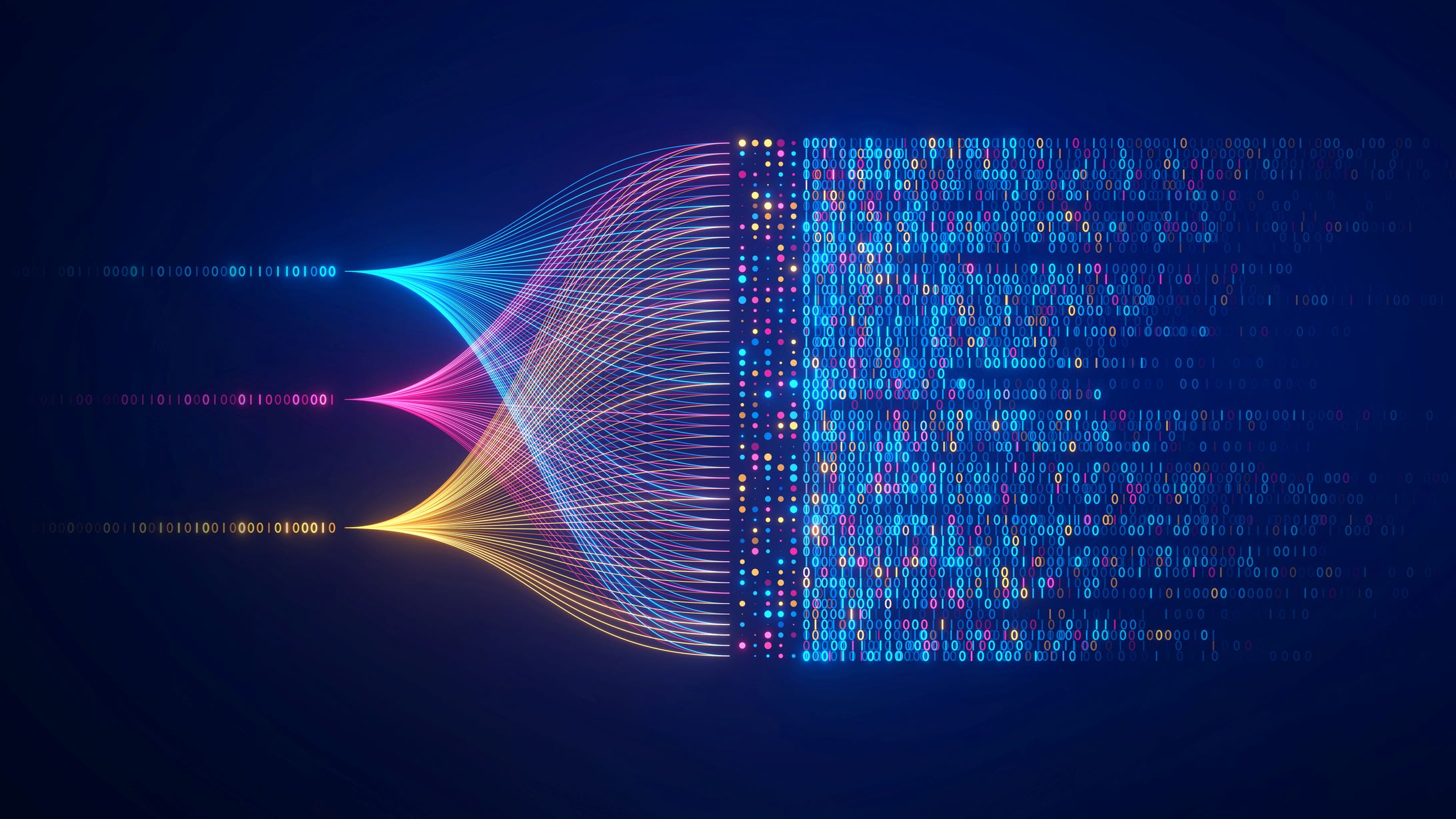
Machine learning (ML) is transforming the healthcare industry, offering innovative ways to improve patient care, optimize decision making, and streamline operations. As a subset of artificial intelligence (AI), machine learning uses algorithms to analyse vast amounts of data, identify patterns, and generate insights that can help healthcare providers make more accurate diagnoses, personalize treatments, and predict outcomes.
What is machine learning in healthcare?
Machine learning refers to the process where computers learn from data without being explicitly programmed. In healthcare, ML algorithms are trained using large datasets, which can include patient records, medical images, genetic information, and more. By learning from this data, the algorithms can detect patterns and make predictions or recommendations, supporting medical decisions in real time.
The power of machine learning lies in its ability to process and analyse data far beyond human capabilities, uncovering trends that may not be immediately apparent. As healthcare systems generate massive amounts of data, ML is an essential tool for translating that information into actionable insights.
Key benefits of machine learning in healthcare
The use of machine learning in healthcare provides several benefits that drive improvements in patient care and operational efficiency:
Enhanced diagnostic accuracy
One of the most significant advantages of machine learning in healthcare is its ability to improve diagnostic accuracy. ML algorithms can analyse complex datasets, such as medical images or lab results, and identify patterns that may be missed by human clinicians. This leads to earlier and more precise diagnoses, which can be critical for conditions like cancer, heart disease, and rare genetic disorders.
For example, ML algorithms trained to detect anomalies in radiological images can assist radiologists in identifying tumours or fractures more quickly and accurately, reducing diagnostic errors and improving patient outcomes.
Predictive analytics for patient outcomes
Machine learning is invaluable when it comes to predictive analytics, which allows healthcare providers to anticipate patient outcomes and adjust treatment plans accordingly. By analysing historical patient data, ML models can predict the likelihood of complications, readmissions, or disease progression. This enables proactive interventions, reducing the need for reactive treatments and improving overall patient care.
For instance, predictive analytics can help identify patients at high risk of developing conditions like sepsis or diabetes, enabling earlier interventions and personalized care plans to mitigate those risks.
Personalized medicine
Machine learning is driving the shift toward personalized medicine, where treatments are tailored to the individual needs of each patient. By analysing genetic, environmental, and lifestyle data, ML algorithms can recommend therapies that are most likely to be effective for a particular patient. This approach minimizes trial and error treatments and reduces the risk of adverse reactions.
Pharmacogenomics, the study of how genes affect a person’s response to drugs, is an area where machine learning plays a crucial role. By analysing genetic data, ML algorithms can predict how a patient will respond to a medication, allowing healthcare providers to choose the most effective drug and dosage.
Optimized clinical workflows
In addition to improving patient care, machine learning can enhance the efficiency of clinical workflows. By automating administrative tasks such as appointment scheduling, billing, and patient record management, ML reduces the burden on healthcare staff, allowing them to focus more on patient care. Machine learning powered chatbots and virtual assistants can also help patients book appointments, answer health related questions, and provide guidance, improving the patient experience.
Predictive maintenance is another area where ML improves workflows. By analysing equipment usage data, machine learning can predict when medical devices or hospital equipment are likely to fail, allowing for timely maintenance and reducing downtime.
Applications of machine learning in healthcare
Machine learning has numerous applications across various areas of healthcare, from diagnostics to drug discovery.
Below are some key applications where ML is making a significant impact:
Remote patient monitoring
Remote patient monitoring is an area where machine learning excels, enabling healthcare providers to track patients’ health data in real-time. Wearable devices, such as smartwatches or sensors, continuously collect data on a patient’s heart rate, blood pressure, glucose levels, and other vital signs. Machine learning algorithms can analyse this data to detect trends or early warning signs of deterioration, allowing for timely interventions.
For example, ML powered remote monitoring can help manage chronic conditions like diabetes by alerting healthcare providers when a patient’s blood sugar levels are out of range. This continuous monitoring improves patient outcomes and reduces hospital readmissions.
Medical imaging and diagnostics
Machine learning is transforming medical imaging by enabling computers to analyse X-rays, MRIs, CT scans, and other types of medical images with remarkable accuracy. By training ML models on thousands of images, these systems can detect abnormalities such as tumours, lesions, and fractures. In some cases, machine learning models can diagnose conditions faster and with greater accuracy than human specialists.
ML based diagnostic tools are also being applied to pathology, helping pathologists analyse tissue samples and identify diseases like cancer more efficiently.
Drug discovery
Drug discovery is a complex and time consuming process, often taking years to develop and test new medications. Machine learning is revolutionizing this process by speeding up drug discovery and reducing costs. ML algorithms can analyze vast amounts of data on chemical compounds and biological targets, identifying potential drug candidates much faster than traditional methods.
Machine learning models can also predict how different drugs will interact with various biological systems, improving the efficiency of preclinical trials and increasing the likelihood of success in clinical trials.
Natural language processing (NLP) in healthcare
Natural language processing (NLP), a subfield of machine learning, is increasingly being used to analyse unstructured data such as doctors' notes, electronic health records (EHRs), and medical literature. NLP algorithms can extract relevant information from these sources, providing insights into patient history, symptoms, and treatment outcomes.
For example, NLP can be used to analyse patient records and identify patterns in symptoms that could indicate a specific disease. It also helps in administrative tasks, such as coding medical records for billing purposes or summarizing lengthy patient reports.
Challenges of machine learning in healthcare
While machine learning holds immense potential, there are challenges that need to be addressed to fully realize its benefits in healthcare:
Data privacy and security
Healthcare data is highly sensitive, and ensuring its privacy and security is paramount. Machine learning models require access to vast amounts of patient data, raising concerns about how this data is stored, shared, and protected. Strict regulations like HIPAA and GDPR must be followed to safeguard patient information.
Bias in algorithms
Another challenge is the potential for bias in machine learning algorithms. If the data used to train models is not representative of diverse patient populations, the resulting predictions and diagnoses may be biased. This can lead to disparities in care, particularly for underrepresented groups. Ensuring diversity in training data and regularly testing algorithms for fairness are essential steps in addressing this issue.
How ProdActive leverages machine learning in healthcare
At ProdActive, we are harnessing the power of machine learning to develop cutting edge healthcare solutions that drive better outcomes for patients and providers. From predictive analytics to personalized care platforms, our team creates AI-driven software that enhances diagnostic accuracy, optimizes workflows, and transforms patient care.
If you're interested in learning how machine learning can revolutionize your healthcare practice or improve patient outcomes, contact ProdActive today.
Let us help you integrate machine learning into your healthcare solutions for a smarter, more efficient future.